The AI Supercycle: Why Compute is the Most Valuable Commodity of the Decade
- Sam Leigh
- Feb 12
- 5 min read
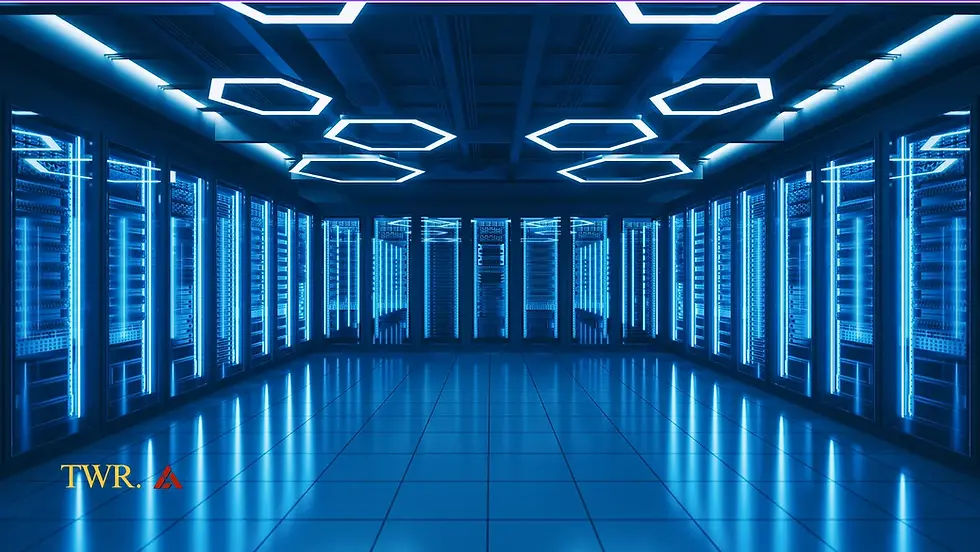
by Sam Leigh | February 12, 2025
Artificial intelligence is no longer just a trend — it is the economic backbone of the future. While generative AI, model wars, and user adoption dominate the headlines, the most significant transformation is unfolding beneath the surface. AI is now constrained not by algorithms, but by compute.
This reality is shaping the power dynamics of AI more than any single breakthrough in neural network architecture. The public remains fixated on the capabilities of OpenAI’s latest ChatGPT update, Google’s Gemini advancements, or Anthropic’s Claude refinements, yet industry insiders understand the real limitation: AI models are only as powerful as the hardware infrastructure that supports them. Compute power, driven by advanced GPUs, next-generation memory architectures, and energy-intensive data centers, is the defining factor in AI’s acceleration.
The AI race is no longer just a competition between model architectures — it is a global infrastructure battle. The companies and nations that control AI compute resources will dictate the next decade of technological and economic dominance.
The Compute Shortage: AI’s Hidden Crisis
Every major AI system today — whether it’s generating search results, optimizing supply chains, detecting financial fraud, or developing pharmaceuticals — relies on accelerated computing. Yet the demand for processing power is increasing at an exponential rate, far outpacing supply.
The problem is structural. AI models are not simply getting better; they are getting exponentially larger and more resource-intensive. GPT-4 required more than ten times the compute power of its predecessor, GPT-3. DeepMind’s AlphaFold-3, which is used for protein structure prediction, demands access to entire supercomputing clusters. And OpenAI’s rumored GPT-5 is expected to require upwards of 100,000 Nvidia H100 GPUs just to train — an astronomical level of compute power.
The situation is even more dire in enterprise AI. Financial institutions, logistics companies, and biotech firms are aggressively deploying AI for mission-critical applications, yet they are finding that access to high-performance computing is becoming the biggest barrier to progress. AI isn’t being limited by innovation — it’s being limited by availability of infrastructure.
The Nvidia Bottleneck and the GPU Arms Race
No company embodies the AI arms race more than Nvidia. The dominance of its GPUs has made it the most critical hardware provider in the AI economy, with its H100 and upcoming B100 accelerators becoming the defining assets in modern computing.
Tech giants such as Microsoft, Google, Meta, and Amazon are engaged in a bidding war over Nvidia’s chips, stockpiling tens of billions of dollars’ worth of AI accelerators. This hoarding has led to severe shortages, making it nearly impossible for smaller firms to gain access to the compute resources needed for large-scale AI training.
The numbers reveal the severity of the situation. Nvidia’s AI-driven revenue skyrocketed from $3 billion to over $40 billion in just three years. The collective investment by Microsoft, Google, and Amazon in AI infrastructure is expected to exceed $250 billion in 2025. Meanwhile, AI data centers are consuming more electricity than entire nations.
And yet, despite this massive investment, supply remains critically constrained. Companies are being forced to redesign AI architectures around hardware limitations, prioritizing efficiency over raw performance. AI model development is increasingly dictated not by theoretical advances, but by what is computationally feasible.
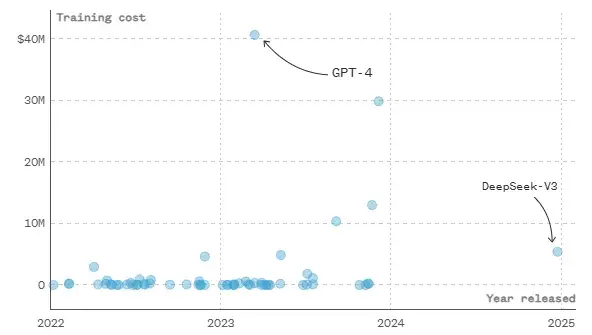
The Power Crisis: AI’s Next Bottleneck
While GPUs remain the most immediate bottleneck, power consumption is rapidly becoming an equally pressing issue. AI systems are voracious consumers of electricity, to the point where the energy cost of operating large models is now a fundamental economic constraint.
Training a single GPT-4-level model requires as much electricity as powering a mid-sized city for months. But training is only one part of the problem — running AI models at scale consumes even more energy over time. AI inference, which refers to the real-time execution of trained models, operates continuously, requiring sustained compute power.
This has led to a wave of direct power agreements between AI firms and energy providers, including nuclear power plants, solar farms, and dedicated AI energy grids. Companies are also investing heavily in custom silicon chips designed for power efficiency, such as Google’s TPUs, Intel’s Gaudi processors, and IBM’s Loihi neuromorphic chips.
Governments, meanwhile, are scrambling to ensure energy resilience. Some nations are already planning dedicated AI power zones, recognizing that the future of AI will be determined by who controls the energy resources required to sustain it.
DeepSeek-V2: A Wake-Up Call, Not a Revolution
In early 2024, China’s AI sector made global headlines with the unveiling of DeepSeek-V2. The model’s most striking feature was not its capabilities — its performance was roughly on par with OpenAI’s GPT-4–01 — but its cost efficiency.
DeepSeek-V2 was trained for just $6 million, a fraction of what was assumed necessary for a high-performance AI system. Some argued this represented a breakthrough in AI efficiency, with China leapfrogging the U.S. in cost-effective training.
However, a deeper analysis reveals a more measured perspective. OpenAI’s GPT-4–01, which was developed over a year prior, is estimated to have cost between $12 million and $20 million to train — a relatively comparable figure. DeepSeek-V2 did not surpass OpenAI’s models in performance; instead, it demonstrated China’s ability to catch up at a fraction of the cost.
This efficiency was achieved through several key optimizations:
Distributed Compute: Running AI workloads on less powerful GPUs at scale.
Custom Silicon R&D: Investing in domestic AI chips to reduce reliance on Nvidia.
Algorithmic Efficiency: Optimizing training techniques to maximize compute power.
The real significance of DeepSeek-V2 lies not in its performance, but in its geopolitical implications. It proved that China could develop competitive AI models despite U.S. restrictions on Nvidia’s high-end chips. While Washington had hoped that cutting China off from advanced AI accelerators would stifle its progress, DeepSeek-V2 sent a clear message: China is finding ways to work around those barriers.
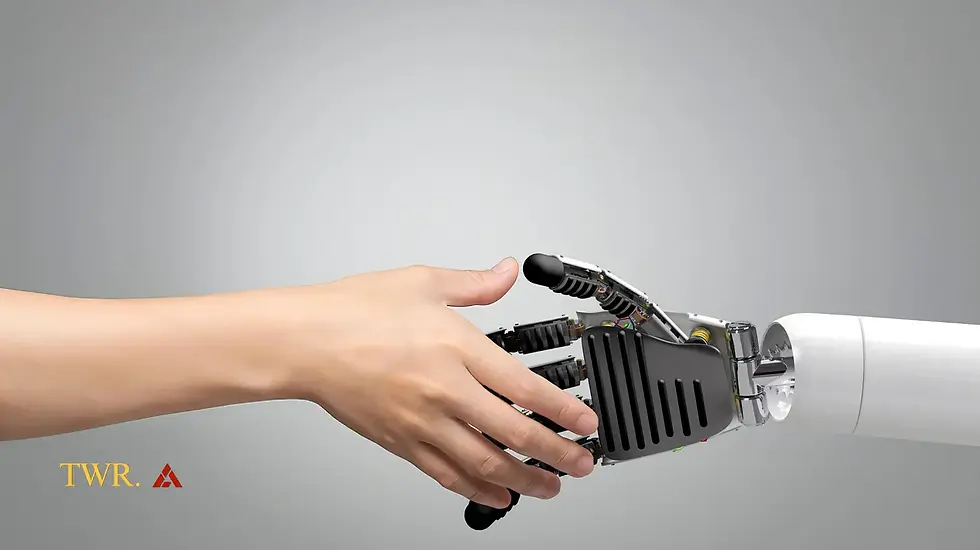
Why Enterprises Need the Right AI Strategy Partner
For businesses, the AI revolution is no longer optional — it is an operational necessity. Yet many companies vastly underestimate the complexity of AI integration.
Some believe they can simply subscribe to an OpenAI API, integrate ChatGPT into their systems, and instantly unlock AI-driven productivity. The reality is far more demanding.
True AI transformation requires a complete restructuring of enterprise architecture — from workflow automation to data governance and long-term scalability.
One of the biggest challenges is navigating the AI platform ecosystem. Each major provider — OpenAI, Google Gemini, Microsoft Copilot — operates in a walled garden. While these platforms offer powerful capabilities, businesses risk vendor lock-in, escalating costs, and data access limitations.
Organizations that fail to develop a platform-agnostic AI strategy risk being locked into suboptimal solutions. Companies need partners who can bridge multiple AI ecosystems, optimize AI models, and future-proof enterprise AI strategy.
Final Thoughts: The AI Supercycle Has Just Begun
The AI revolution is not a one-time event — it is a decades-long transformation that will reshape industries and redefine competitive advantages. Those who invest wisely in compute infrastructure, workforce readiness, and strategic AI deployment will shape the next era of business.
The rest? They will struggle to keep pace.
The companies that control compute, energy, and strategy will dominate the next decade of AI.
The only question left is: Who is ready for what comes next?
Commentaires